Invited Talks
-
Ashok Kumar
Information Geometric Approach to Simple and Robust Linear Regression Problems -
Pooja Vyavahare
Distributed Learning with Partial Information Sharing -
Mrinal Kumar
High Rate Multivariate Polynomial Evaluation Codes -
Myna Vajha
Two Way Generalization of a Binary Burst Correcting Code -
Jithin Ravi
On the Second-Order Asymptotics of the Hoeffding Test and Other Divergence Tests -
Arpan Chattopadhyay
Inverse Particle and Ensemble Kalman Filters
Ashok Kumar
Information Geometric Approach to Simple and Robust Linear Regression Problems
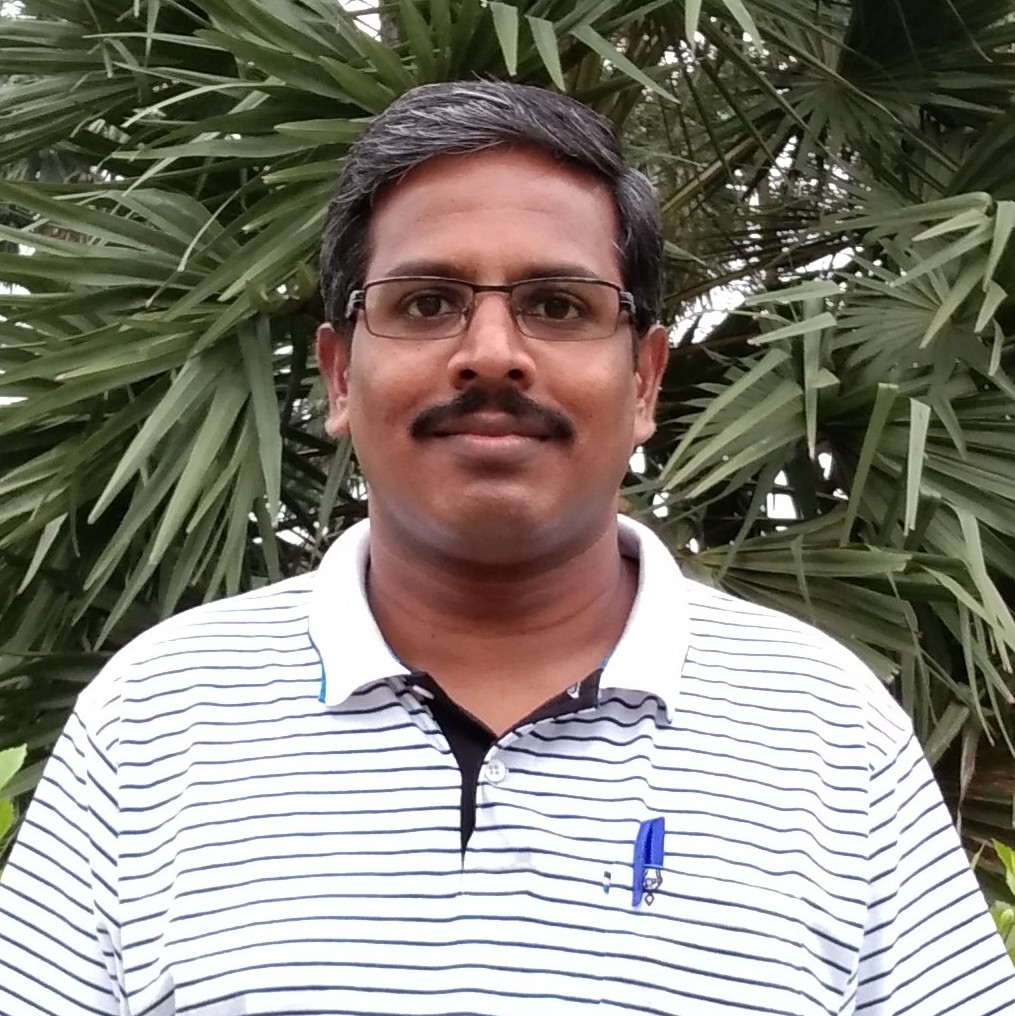
It is generally known that maximum likelihood estimation (MLE) and least squares estimation (LSE) yield the same estimate for regression coefficients in linear regression models under normality assumption of errors. This further demonstrates that the linear regression problem can also be solved by an information geometric approach by minimizing the Kullback-Leibler divergence between two multivariate normal densities. The same idea extends to weighted least squares estimation and generalized least squares estimation in the context of linear regression. We will see that this further extends to another couple of Student-t distribution and minimum power estimator if the power defining the estimator is the same as the degrees of freedom of the t-distribution.
M. Ashok Kumar received his bachelor’s and master’s degrees in Mathematics from the Manonmaniam Sundaranar University, Tirunelveli, in 1999 and 2001 respectively. He was a lecturer in Mathematics in various educational institutions during 2001 - 2007. He obtained a CSIR-UGC fellowship and joined for a Ph.D programme in the department of Electrical Communication Engineering of the Indian Institute of Science, Bangalore in 2008. He completed his Ph.D in October 2014. Subsequently, he was a ‘Visiting Scientist’ at the Stat-Math Unit of the Indian Statistical Institute, Bangalore during December 2014 and May 2015 and a postdoctoral fellow at the department of Electrical Engineering of the Technion-Israel Institute of Technology during May 2015 and December 2015. He was with the discipline of Mathematics at IIT Indore from January 2016 to May 2018. He has been with IIT Palakkad since June 2018.
Pooja Vyavahare
Distributed Learning with Partial Information Sharing
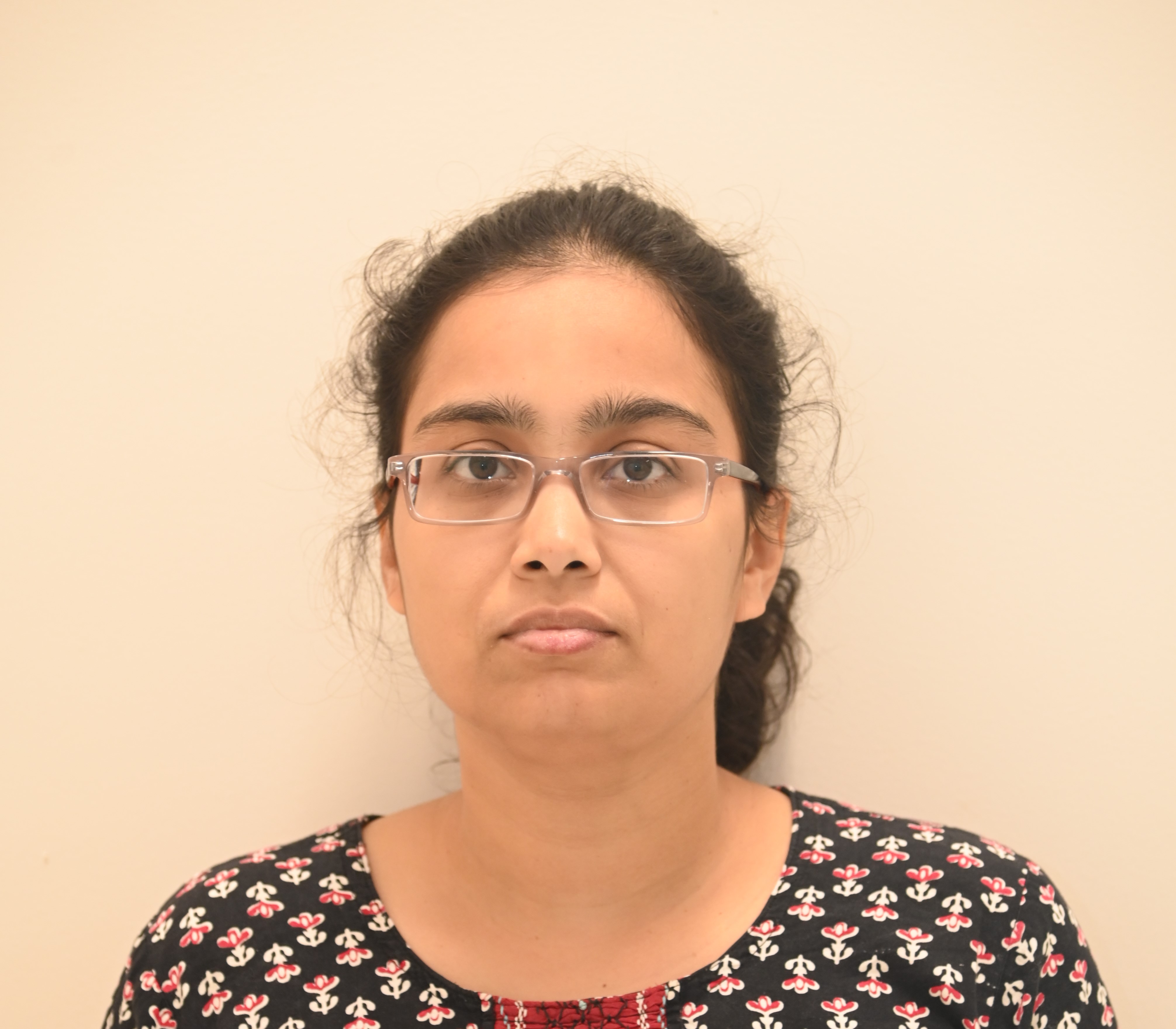
In this talk, we will present the distributed hypothesis testing (distributed learning) problem in multi-agent networks wherein each agent observes some private signals generated by an unknown hypothesis and then tries to learn the hypothesis by exchanging information with neighbours. Non-Bayesian update algorithms in which agents share only their belief vector on the hypothesis set at a time are well known in the literature. We will present a communication-efficient distributed algorithm for the distributed learning in which agents share quantized belief on only one hypothesis at a time. We show that even with partial information sharing, agents learn the true underlying hypothesis almost surely subject to some network identifiability condition. We show that randomized communication is necessary for true learning to happen on the network with partial sharing. In the second half of the talk, we will extend the distributed learning in trust-mistrust networks and present some results for structurally balanced networks.
Based on joint work with P Raghavendra Rao.
Pooja Vyavahare is an Assistant Professor at Department of Electrical Engineering, Indian Institute of Technology at Tirupati since 2019. Before joining IIT Tirupati, she was a postdoctoral fellow with the Coordinated Science Laboratory at University of Illinois at Urbana-Champaign. She obtained her Ph.D from the Department of Electrical Engineering, IIT Bombay in 2016. Subsequently, she was briefly with CSE, IIT Delhi as a postdoc and later at the School of Computing and Electrical Engineering, IIT Mandi as a DST-INSPIRE faculty. Her research interests include distributed algorithms on networks and network economics.
Mrinal Kumar
High Rate Multivariate Polynomial Evaluation Codes
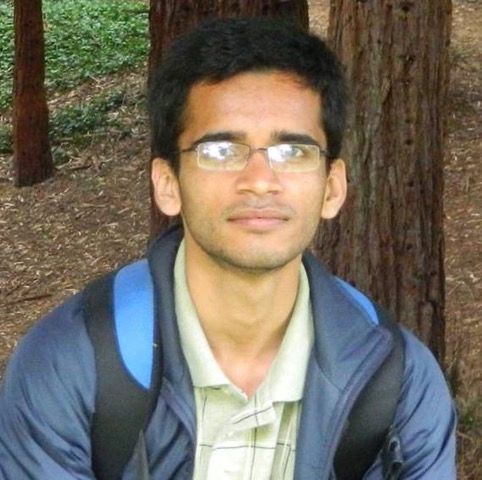
The classical Reed-Muller codes over a finite field are based on evaluations of -variate polynomials of degree at most over a product set , for some . Because of their good distance properties, as well as the ubiquity and expressive power of polynomials, these codes have played an influential role in coding theory and complexity theory. This is especially so in the setting of being where they possess deep locality properties. However, these Reed-Muller codes have a significant limitation in terms of the rate achievable — the rate cannot be more than .
In this talk, I will discuss a recent work where we give two explicit constructions of multivariate polynomial evaluation codes which overcome this rate limitation. More concretely, we give explicit evaluation domains in on which evaluating -variate polynomials of degree at most gives a good code. For constant , these new codes have constant relative distance and rate for any . We give two such constructions of evaluation domains, the first based on combinatorial ideas, and the second a bit more algebraic. The underlying structure of these point sets also yields clean unique decoding algorithms for these codes up to half their minimum distance, as well as endows the second construction with some strong and surprising locality properties.
Based on joint work with Swastik Kopparty and Harry Sha.
Mrinal Kumar is a faculty member in Computer Science at the Tata Institute of Fundamental Research in Mumbai. His research interests are primarily in Algebraic Complexity, Error Correcting Codes and Algebra & Computation. He did his PhD in Computer Science at Rutgers University, and spent a few years as an assistant professor at IIT Bombay before moving to TIFR.
Myna Vajha
Two Way Generalization of a Binary Burst Correcting Code
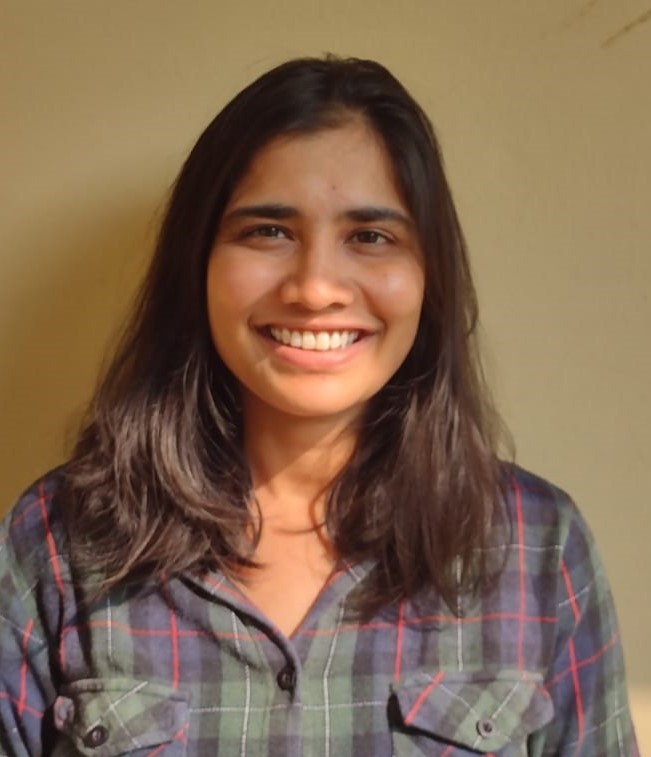
In this talk, we will present two generalizations of a binary code introduced by Hollmann and Tolhuizen (HT) that can correct any burst erasure including a wrap-around burst. The HT code is constructed by recursively concatenating identity matrices of appropriate sizes, either column-wise or row-wise, depending on the parameters. HT codes were initially introduced in the context of constructing streaming codes capable of recovering from a burst of size $b$ within a delay constraint of $\tau$.
The first generalization of HT code allows us to construct a streaming code that can recover from either a random erasure of size $a$ or a burst erasure of size $b$ within a delay constraint of $\tau$. This construction is presented by describing the parity-check matrix that could be thought of as placing together the pieces of a jigsaw puzzle. Specifically, the bottom-left submatrix of length $(a \times (\tau+1))$ is a parity check matrix of an MDS code and a sub-matrix of size $(b-a) \times (\tau-b)$ appearing in the top is recursively constructed by concatenating identity matrices row and column wise. The column-wise expansion introduces $a$ zero columns before concatenating the identity matrix.
The second generalization of the HT code is relevant in the context of a three-node relay setting, where both links experience at most one burst erasure of size $b$. Burst erasure in the source-relay link can lead to message symbols being transmitted out of order at the relay. This also imposes stricter delay requirements on the shifted message symbols. To address this, we permute the columns of the generator matrix of the HT code and reset some coefficients to zero. We show that this modification ensures that the resultant code, defined by the adjusted matrix, satisfies the stricter delay requirement.
Myna Vajha is an Assistant Professor at the EE department, IIT Hyderabad. She received BTech. (2011) and M.Tech. (2013) degrees from IIT Kharagpur and University of Southern California respectively, and the Ph.D. degree from IISc Bangalore in 2020. Post PhD, Myna worked on physical layer algorithms for WiFi at Qualcomm Wireless R&D, Bangalore. She has prior industry experience working on networking layer aspects at Ericsson, San Jose as a Systems architect and as a software engineer at Qualcomm, Hyderabad.
Jithin Ravi
On the Second-Order Asymptotics of the Hoeffding Test and Other Divergence Tests
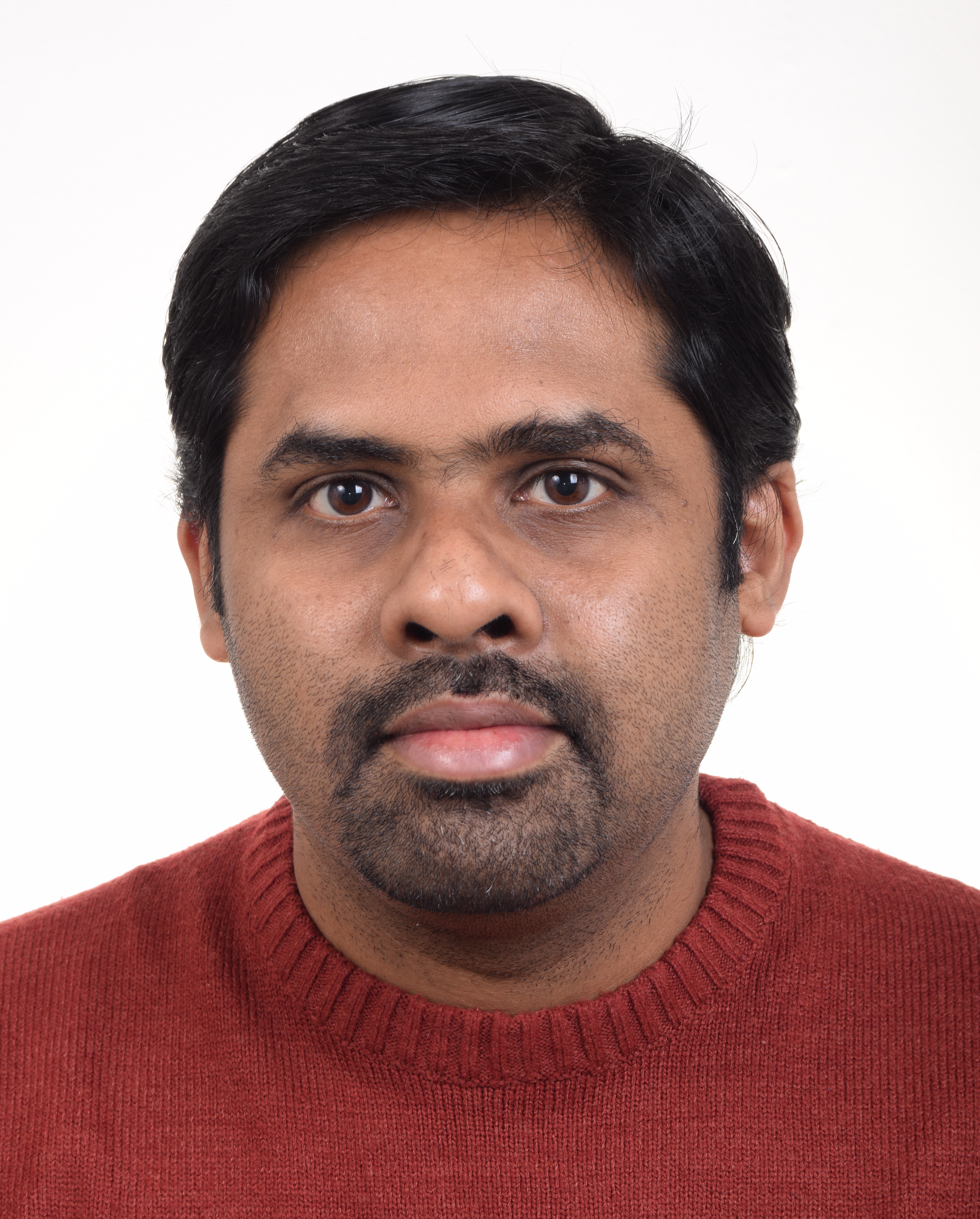
Consider a binary statistical hypothesis testing problem, where n independent and identically distributed random variables $Z^{n}$ are either distributed according to the null hypothesis $P$ or the alternative hypothesis $Q$, and only $P$ is known. A well-known test that is suitable for this case is the Hoeffding test, which accepts $P$ if the Kullback-Leibler (KL) divergence between the empirical distribution of $Z^{n}$ and $P$ is below a given threshold. In this talk, I will present our characterization of the first- and second-order terms of the type-II error for a fixed type-I error for this test, as well as for divergence tests, where the KL divergence is replaced by a general divergence. Our results demonstrate that, irrespective of the divergence, the divergence test achieves the first-order term of the Neyman-Pearson test, which is the optimal test when both $P$ and $Q$ are known. In contrast, the second-order term of the divergence test is strictly worse than that of the Neyman-Pearson test. Interestingly, the divergence test may outperform the Hoeffding test for some alternative hypotheses $Q$.
Jithin Ravi is an assistant professor in the Department of Electronics and Electrical Communication Engineering at the Indian Institute of Technology Kharagpur. He received his B.Tech. degree in electronics and communication from the University of Kerala, Thiruvananthapuram, India, in 2006, and the M.Tech. degree and Ph.D. in electrical engineering from the Indian Institute of Technology Bombay, Mumbai, India, in 2017. From 2006 to 2008, he worked as a Software Engineer at Infosys Technologies Ltd. He did his first Post-Doctoral fellowship at the Tata Institute of Fundamental Research, Mumbai, in 2017. He did his second post-doctoral fellowship with the Signal Theory and Communications Department, Universidad Carlos III de Madrid, Leganés, Spain, from 2018 to 2021. His research interests include information theory, wireless communications, information-theoretic security, and statistical inference.
Arpan Chattopadhyay
Inverse Particle and Ensemble Kalman Filters
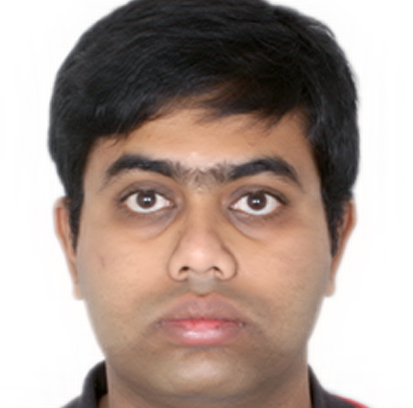
Recent advances in inverse cognition and counter-adversarial systems have led to the development of inverse Bayesian filters. In this setting, a forward Bayesian filter is used by a cognitive adversary to track its target of interest. Subsequently, an inverse filter is utilized to infer the adversary’s estimation of the target or defender’s state. Previous studies have addressed this inverse filtering problem by introducing inverse Kalman filter (I-KF), inverse extended KF (I-EKF), and inverse unscented KF (I-UKF) methods. However, these inverse filters typically assume additive Gaussian system models and/or rely on local approximations of non-linear dynamics at the state estimates. Consequently, their practical application is limited. In contrast to prior works, this work adopts a global filtering approach and presents the development of an inverse particle filter (I-PF). The particle filter framework employs Monte Carlo (MC) methods to approximate arbitrary posterior distributions. Moreover, under mild system-level conditions, the proposed I-PF demonstrates convergence to the optimal inverse filter. Additionally, we explore MC techniques to approximate Gaussian posteriors and introduce the inverse Gaussian particle filter (I-GPF) and inverse ensemble KF (I-EnKF) methods. Using the recursive Cramer-Rao lower bound and non-credibility index (NCI), our numerical experiments for several different applications demonstrate the estimation performance and time complexity of the proposed filters.
Arpan Chattopadhyay completed his B.E. from Jadavpur University, Kolkata in 2008, and M.E. and PhD from Indian Institute of Science, Bangalore, in 2010 and 2015, respectively. Currently he is serving as an assistant professor at the Electrical Engineering department, IIT Delhi. Previously, he had held postdoc positions at INRIA, Paris, France, and the University of Southern California, Los Angeles, USA. His research interests include wireless communication and networks, signal processing and online learning.